AEJPSAsian European Journal of Probability and Statistics
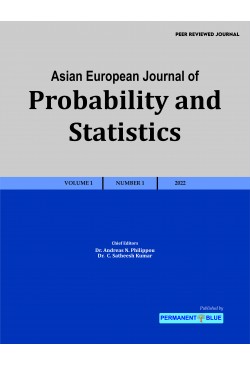
Peer Reviewed Journal
Peer Reviewed Journal
A direct construction of the Wiener measure on C[0,∞)
A revisit on the survival analysis of two popular data sets via nonparametric and semi-parametric methods: New results with discussion
Limiting Behavior of Two M/M/1 Queues Sharing a Common Waiting Room
Asymmetric gamma generalized curved normal distribution
An optimal replacement policy under variable shocks and different patterns of self-healing
Can Additive Processes be Stationary?